Co-authored by Yihua Liao and Yi Zhang
You have probably heard of how AI technology is used to recognize cats, dogs and humans in images, a task known as image classification. The same technology that identifies a cat or dog – can also identify sensitive data (such as identification cards and medical records) in images traversing your corporate network. In this blog post, we will show you how we use convolutional neural networks (CNN), transfer learning, and generative adversarial networks (GAN) to provide image data protection for Netskope’s enterprise customers.
Image Data Security
Images represent over 25% of the corporate user traffic that goes through Netskope’s Data Loss Prevention (DLP) platform. Many of these images contain sensitive information, including customer or employee personally identifiable information (PII) (e.g., pictures of passports, driver’s licenses, and credit cards), screenshots of intellectual property, and confidential financial documents. By detecting sensitive information in images, documents, and application traffic flows, we help organizations comply with compliance regulations and protect their assets.
The traditional approach to identifying sensitive data in an image has been to use optical character recognition (OCR) to extract text out of the image. The extracted text is then used for pattern matching. This technology, though effective, is resource-intensive and delays detection of security violations. OCR also has difficulties identifying violations in low-quality images. In many cases, we only need to determine the classification of the input image. For example, we would like to find out whether an image is a credit card or not, without knowing the 16-digit card number and other details in the image. Machine learning-based image classification is an ideal choice for that because of its accuracy, speed and ability to work inline with granular policy controls. We can also combine image classification with OCR to generate more detailed violation alerts.
CNN and Transfer Learning
Deep learning and convolutional neural networks (CNN) were a huge breakthrough in image classification in the early 2010s. Since then, CNN-based image classification has been applied to many different domains, including medicine, autonomous vehicles, and security, with accuracy close to that of humans. Inspired by how the human visual cortex works, a CNN is able to effectively capture the shapes, objects and other qualities to better understand the contents of the image. A typical CNN has two parts (depicted in the chart below):
- The convolutional base, which consists of a stack of convolutional and pooling layers. The main goal of the convolutional base is to generate features from the image. It builds progressively higher-level features out of an input image. The early layers refer to general features, such as edges, lines, and dots in the image. Meanwhile, the latter layers refer to task-specific features, which are more human interpretable, such as the logo on a credit card, or application windows in a screenshot.
- The classifier, which is usually composed of fully connected layers. Think of the classifier as a machine that sorts the features identified in the convolutional base. The classifier will tell you if the features identified are a cat, dog, drivers license, or X-ray.
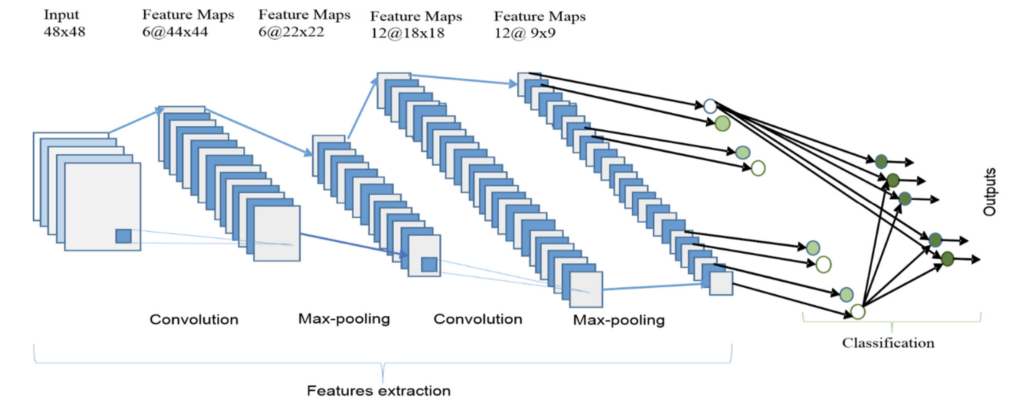
You may need millions of labeled images to train a CNN from scratch in order to achieve state-of-the-art classification accuracy. It is not trivial to collect a large number of images with proper labels, especially when you are dealing with sensitive data such as passports and credit cards. Fortunately, we can use transfer learning, a popular deep learning technique, to train a neural network with just hundreds or thousands of training samples. With transfer learning, we can leverage an existing convolutional neural network (e.g., ResNet or MobileNet) that was trained on a large dataset to classify other objects, and tweak it to train with additional images. Transfer learning allows us to train a CNN image classifier with a limited dataset and still achieve good performance while significantly reducing the training time.
Synthetic Training Data Generation
It’s very challenging to acquire real images for the sensitive categories we are interested in. To increase the amount and diversity of the training dataset and further improve the accuracy of CNN classifiers, we use generative adversarial networks (GAN) to generate synthetic training data. The basic idea of a GAN is to create two neural networks (high-level architecture diagram below), which compete against each other. One neural network, called the generator, generates fake data, while the other, the discriminator, evaluates them for authenticity. The goal is to generate data that is similar to the training data and fool the discriminator.
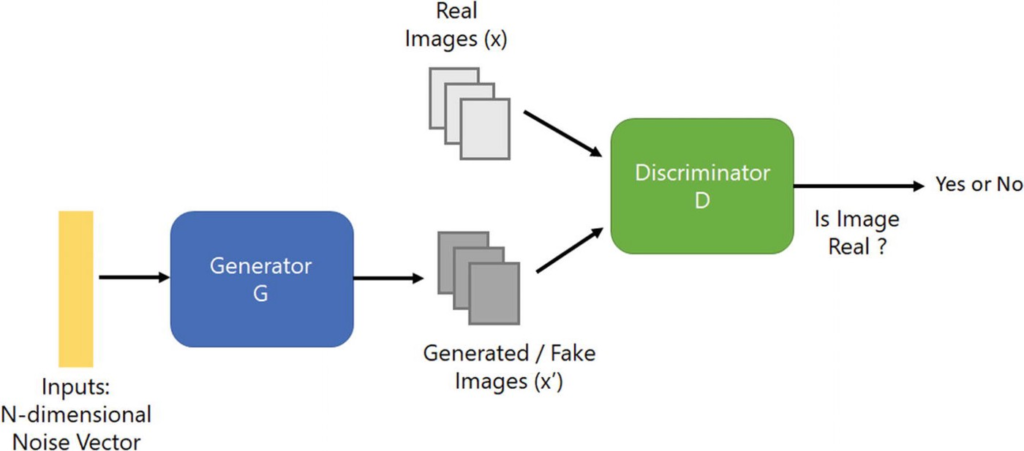
With a GAN, we are able to synthesize photorealistic images with varying degrees of change in rotation, color, blurring, background, and so on. Here are a few examples of the synthetic images:
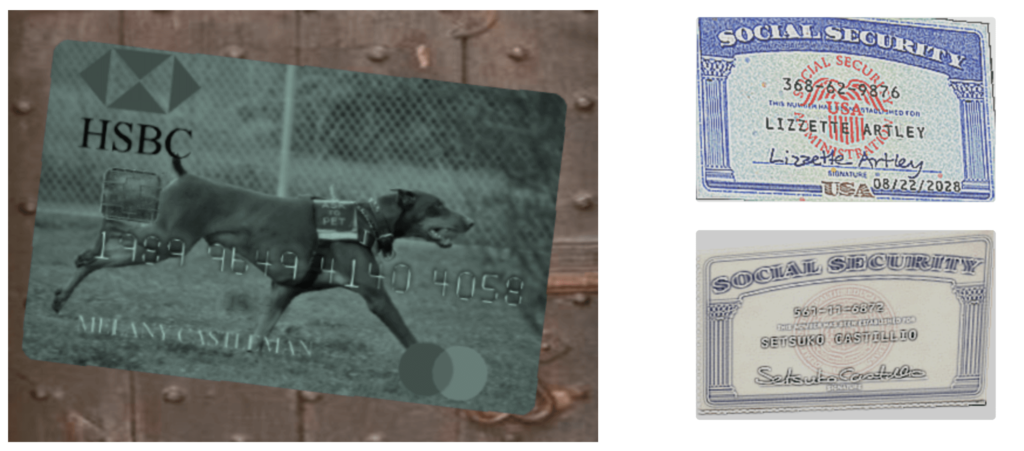
Netskope’s Inline DLP Image Classifiers
At Netskope, we have developed CNN-based image classifiers, as part of our Next Gen SWG and cloud inline solutions covering managed apps, unmanaged apps, custom apps, and public cloud service user traffic. The classifiers are able to accurately identify images with sensitive information, including passports, driver’s licenses, US social security cards, credit cards and debit cards, fullscreen and application screenshots, etc. The inline classifiers provide granular policy controls in real-time.
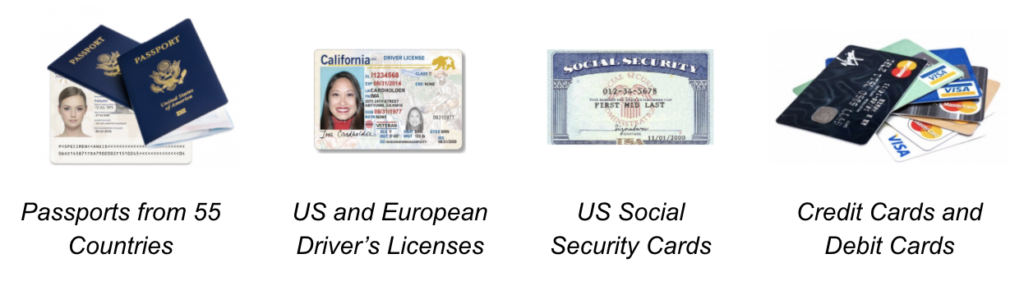
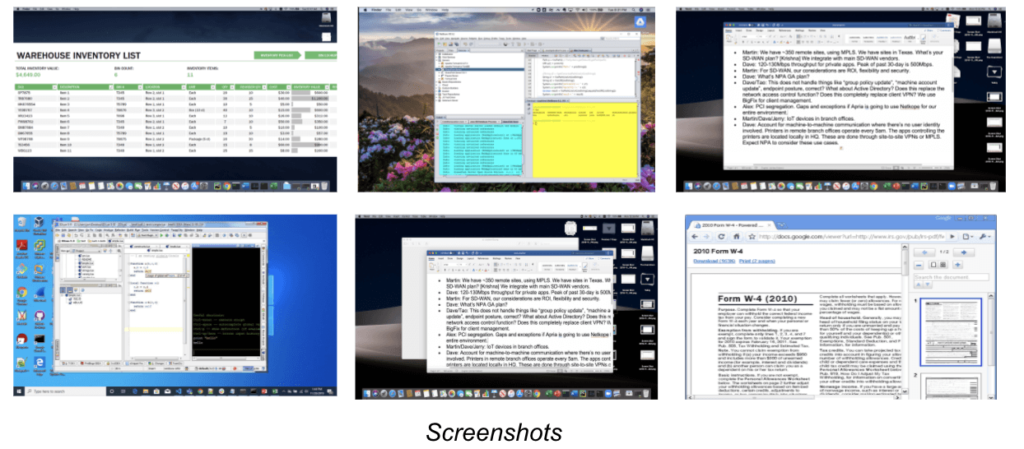
Future Work
At Netskope, we are actively expanding our portfolio of inline image classifiers with the latest computer vision technology. We also have the capability to train custom classifiers and identify new types of images that our customers are interested in classifying. If your organization has unique assets that may be shared in images and you’d like to protect those assets, please contact us at [email protected] to learn more.